•8 min read
Multimodal Monday #8: Faster Systems, Faster Impact
A weekly pulse on everything multimodal—models, data, tools & community.
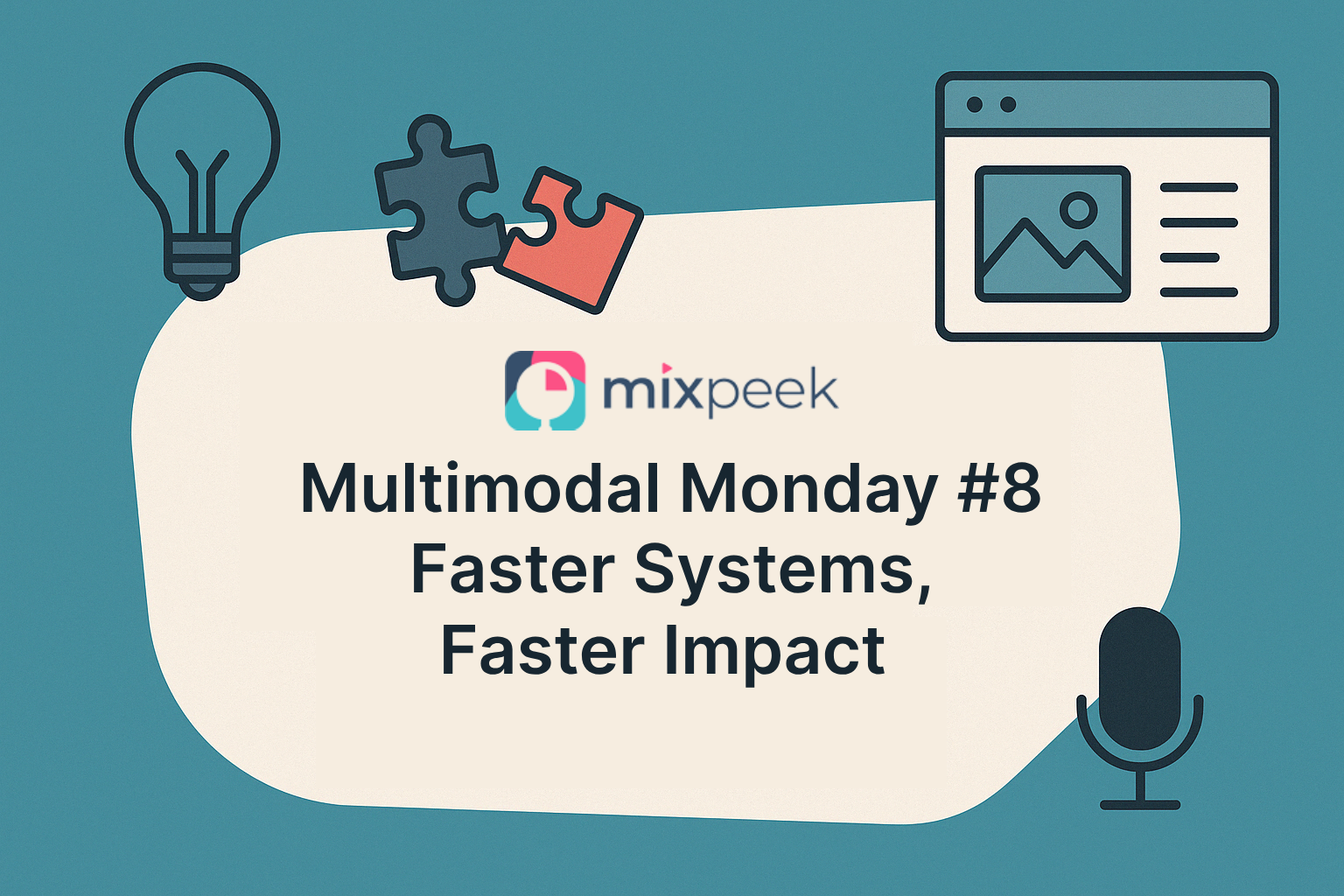
Multimodal Monday
📢 Quick Takes (TL;DR)
- Speed Becomes Competitive Edge - From LTX-Video's 9.5-second generation to Lightning V2's 100ms speech synthesis, multimodal systems are racing to real-time performance.
- Efficiency Without Compromise - Quantized models, distillation techniques, and lightweight architectures show how multimodal capabilities can be delivered with dramatically reduced resource requirements.
- Multimodal Systems Move from Lab to Real World - This week's standouts show multimodal AI entering critical infrastructure, with Walmart preparing for AI shopping agents and New York State deploying computer vision across government agencies, signaling a shift from experimental to production-ready multimodal applications.
🧠 Research Highlights
- DanceGRPO: Reinforcement Learning Meets Visual Generation: A new research paper introduces DanceGRPO, the first unified framework to adapt Group Relative Policy Optimization (GRPO) to visual generation paradigms. This framework applies reinforcement learning techniques across multiple visual generation tasks, including diffusion models, rectified flow, image generation, video generation, and 3D generation.
Why It Matters: This research demonstrates how reinforcement learning can enhance visual generation capabilities across different modalities, potentially improving the quality and controllability of generated visual content in multimodal systems that need to produce diverse visual outputs from unified understanding. Paper | Project Page | Tweet - ByteDance WildDoc VQA Dataset: ByteDance has released WildDoc, a new Visual Question Answering (VQA) dataset designed specifically to evaluate the document understanding capabilities of Vision-Language Models (VLMs) in real-world scenarios. This dataset focuses on challenging real-world document examples with complex layouts, varied formatting, and diverse content types.
Why It Matters: By providing a more realistic evaluation framework for document understanding systems, WildDoc could drive significant improvements in multimodal indexing and retrieval systems that need to process, understand, and extract information from complex document images in practical applications. GitHub | Tweet - Meta CATransformers Research: Meta AI has introduced CATransformers, a carbon-driven neural architecture and system hardware co-design framework. Using this framework, researchers have discovered greener CLIP (Contrastive Language-Image Pre-training) models that achieve an average of 9.1% reduction in total lifecycle carbon emissions while maintaining accuracy, with some variants reaching up to 17% reduction.
Why It Matters: This research addresses the growing environmental concerns around large-scale AI systems, particularly multimodal models that tend to be computationally intensive, potentially influencing how future multimodal systems are designed and deployed to be more sustainable at scale. Paper | Tweet - HQ-SAM Zero-Shot Segmentation: HQ-SAM (High-Quality Segment Anything Model) has gained new attention with its integration into Hugging Face Transformers. Building upon the original SAM, HQ-SAM produces higher-quality segmentation masks without requiring additional training on new datasets, achieving consistent performance gains across a wide range of zero-shot segmentation tasks.
Why It Matters: High-quality segmentation is fundamental for many vision-language tasks, including object-centric reasoning, visual question answering, and fine-grained image understanding, making this improved boundary precision valuable for multimodal systems that need to accurately identify and process specific visual elements within complex scenes. GitHub | Paper | HuggingFace Docs | Tweet
- Intelligent Document Processing Leaderboard: A new Intelligent Document Processing (IDP) Leaderboard has created a unified benchmark for document understanding tasks. This comprehensive benchmark evaluates multimodal AI systems on various document processing capabilities including OCR, Key Information Extraction (KIE), document classification, visual question answering, table extraction, and more.
Why It Matters: Document understanding is a key multimodal task requiring systems to process both visual and textual information simultaneously, and this standardized evaluation framework could accelerate improvements in multimodal models for document processing, enhancing systems that index, retrieve, and understand information from document images. Website | Tweet
🛠️ Tools & Techniques
- miniCoil Lightweight Sparse Neural Retriever: Qdrant has released miniCOIL, a lightweight sparse neural retriever. This new approach to information retrieval combines the efficiency of traditional term-based methods with the semantic understanding capabilities of neural networks, built on top of the proven BM25 algorithm but enhanced with neural understanding of word meanings.
Why It Matters: Efficient retrieval is critical for multimodal systems that need to quickly search through large collections of content, and the ability to understand semantic relationships while maintaining computational efficiency improves multimodal indexing and retrieval systems that handle text components alongside other modalities. Tweet | Details

- Alibaba Wan2.1-VACE Video Creation and Editing Model: Alibaba has released Wan2.1-VACE (Video All-in-one Creation and Editing), a comprehensive open-source model for video creation and editing. This model supports video creation from various input formats including text, images, and videos, while also enabling sophisticated editing capabilities.
Why It Matters: The integration of multiple video-related tasks into a unified model simplifies multimodal indexing and retrieval systems that need to process and understand video content, potentially streamlining workflows that involve both video generation and manipulation based on multimodal inputs. GitHub | Paper | Project Page | Tweet
- PixVerse V4.5 Video Generation: PixVerse has released version 4.5 of their AI video generation model, introducing several significant enhancements including over 20 cinematic camera control options, multi-element reference and fusion capabilities, and improved handling of complex movements and transitions.
Why It Matters: The growing sophistication of controllable video generation enables more precise and customizable visual content creation within multimodal systems, while the ability to fuse multiple visual references enhances multimodal retrieval systems that need to generate composite visual content based on diverse inputs. GitHub | Replicate | Tweet - FastVideo V1 Framework: Hao AI Lab has released FastVideo V1, a unified framework for accelerating video generation. This framework addresses one of the key challenges in video generation: computational intensity and slow processing times, offering a simple, consistent Python API that works across popular video generation models.
Why It Matters: As one of the most computationally intensive multimodal tasks, video generation benefits significantly from frameworks that accelerate this process, potentially enabling more responsive multimodal systems that incorporate video generation capabilities for interactive applications where generation speed is critical. GitHub | PyPI | Project Page | Tweet - LTX-Video 13B Distilled Models: Lightricks has released new distilled and quantized versions of their LTX-Video 13B model. The new distilled model dramatically reduces generation time, reportedly producing high-definition videos in as little as 9.5 seconds on high-end hardware and requiring only 4-8 diffusion steps compared to the 25+ steps typically needed by other models.
Why It Matters: Optimization techniques like distillation make computationally intensive multimodal tasks more efficient, enabling more responsive and resource-friendly multimodal systems that incorporate video generation capabilities, particularly valuable for applications requiring near real-time visual content creation. Tweet
- Alibaba Quantized models of Qwen2.5-Omni-7B: Alibaba has released quantized versions of their Qwen2.5-Omni-7B multimodal model. The Qwen2.5-Omni is an end-to-end multimodal model capable of understanding text, images, audio, and video inputs while generating text and speech outputs, with the quantized versions offering improved efficiency while maintaining performance.
Why It Matters: More efficient deployment of comprehensive multimodal capabilities is crucial for systems that need to process multiple input modalities simultaneously, particularly on devices with limited resources, making advanced multimodal understanding more accessible across a wider range of deployment scenarios. HuggingFace | GitHub | Paper | Tweet - Manus Image Generation Capabilities: Manus AI has introduced new image generation capabilities to its AI agent platform. This feature allows Manus to generate images based on detailed plans it creates, first analyzing task requirements, developing a conceptual plan, and then executing the generation with specific intent.
Why It Matters: The integration of reasoning and planning with visual generation enhances multimodal systems that need to both understand context and generate appropriate visual content in response, demonstrating a more sophisticated approach to visual content creation that considers broader contextual requirements. Tweet - Lightning V2 TTS Model: Smallest.ai has released Lightning V2, described as "the fastest TTS model" with just 100ms latency (time to first byte). This text-to-speech model features ultra-low latency enabling near real-time speech generation, support for 16 languages, and custom voice options for personalization.
Why It Matters: Audio generation is an important component of comprehensive multimodal systems, and the ability to generate speech with minimal delay enhances multimodal interfaces that need to provide both visual and auditory information simultaneously, improving user experience in applications that combine multiple modalities. Tweet
🏗️ Real-World Applications
- Walmart Prepares for AI Shopping Agents: Walmart is gearing up for a future where AI agents, not humans, do the shopping. The retail giant is exploring how to make its products attractive to autonomous AI agents that will handle shopping tasks on behalf of consumers, from replenishing household essentials to selecting the best products based on personal preferences.
Why It Matters: This shift represents a fundamental change in how multimodal AI systems will interact with e-commerce, requiring retailers to optimize for algorithmic buyers rather than human visual perception, potentially transforming how product information is structured, priced, and presented across digital platforms. Article - Remark Holdings and Google Public Sector Deploy Computer Vision in New York: Remark Holdings is collaborating with Google Public Sector to implement advanced computer vision AI technology across New York State government agencies. Through a two-year Enterprise Cloud Services Agreement, this partnership will enhance the state's ability to process visual data for infrastructure monitoring, public safety, and healthcare delivery.
Why It Matters: This large-scale deployment demonstrates how multimodal vision systems are moving beyond experimental applications into critical government infrastructure, creating real-world impact through improved public services and establishing patterns for how computer vision can be integrated into complex organizational environments. Press Release
📈 Trends & Predictions
- Unified Frameworks with Domain Personalization: This week's developments highlight a significant trend toward unified frameworks that seamlessly integrate multiple modalities and tasks. DanceGRPO's application of reinforcement learning across different visual generation paradigms, recent models like OmniEmbed with unified embeddings across text, images, audio, and video, and Wan2.1-VACE's all-in-one approach to video creation all point to a future where multimodal AI systems are less siloed and more holistically designed.
The most powerful unified frameworks are those that also enable personalization for niche use cases. As multimodal systems mature, we're seeing a dual trend: broad unification of capabilities alongside specialized adaptability for domain-specific applications. This balance is crucial for multimodal indexing and retrieval systems that must serve both general-purpose needs and highly specialized vertical applications like healthcare, legal, or creative industries. - The Race to Real-Time Efficiency: As multimodal models grow in capability, a parallel revolution in efficiency is making them faster, smaller, and more accessible. This week's releases demonstrate a clear industry-wide focus on optimizing multimodal systems for real-world deployment constraints.
The most striking examples include LTX-Video's distilled model generating high-definition videos in as little as 9.5 seconds, Lightning V2's 100ms latency for text-to-speech, and Whisper Large v3 Turbo running 5.4 times faster than its predecessor. These advances are achieved through various techniques: model distillation, quantization, architectural innovations, and specialized frameworks like FastVideo.
These efficiency gains are particularly valuable for personalized, niche applications where specialized multimodal systems must operate within tight resource constraints. By making multimodal AI more efficient, these advances enable the deployment of tailored solutions for specific industries and use cases that previously couldn't justify the computational expense.
🧩 Community + Shoutouts
- Demo of the Week - SmolVLM Realtime Demo: A demonstration of SmolVLM running in real-time showcases the efficiency and speed of this lightweight vision-language model, highlighting advances in making multimodal AI more accessible on consumer hardware. Tweet
- Training vLLM to Solve CAPTCHAs with RL: Researcher Brendan Hogan demonstrated training a vision-language model to solve CAPTCHAs using reinforcement learning, showing how multimodal models can be fine-tuned for specific visual reasoning tasks through RL techniques. Tweet
- Unhinged Fortnite AI Vader with James Earl Jones Voice: Epic Games has implemented an AI version of Darth Vader in Fortnite that uses the voice of original actor James Earl Jones, showcasing the application of voice cloning technology in gaming to create more authentic character experiences. Though Epic Games may want to add some guardrails. Tweet
Mixpeek helps teams deploy production-ready multimodal systems—providing the infrastructure for efficient, real-time multimodal applications that deliver real-world impact. Contact us to get started
Join the Discussion
Have thoughts, questions, or insights about this post? Be the first to start the conversation in our community!
Start a Discussion